Imagine a world where bridges self-repair, batteries charge in mere seconds, and medical implants integrate seamlessly with human tissue. It might sound like the stuff of science fiction, but what if I told you that the next wonder material capable of making these dreams a reality could be created not by a team of scientists in lab coats, but by an artificial intelligence algorithm crunching astronomical amounts of numbers in some data center?
We are approaching a frontier where AI is meeting material science, a collaboration that could fundamentally alter how we interact with the world around us. In this article, we’ll explore the staggering potential and the real-world applications of AI-designed materials and just how close we are to that next wonder material.
Table of Contents
Can Artificial Intelligence Create The Next Wonder Material?
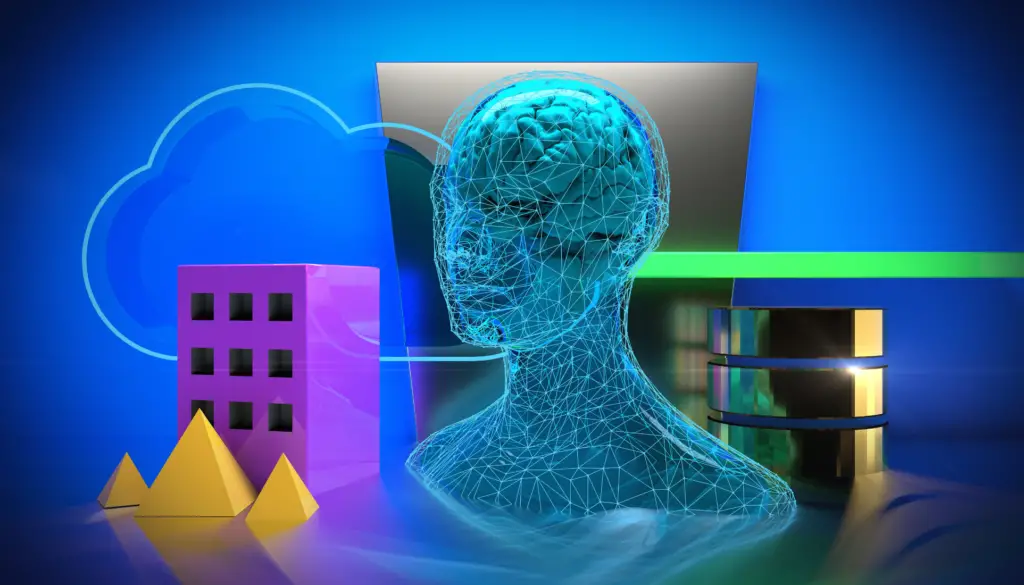
Absolutely, AI has already shown incredible promise in creating new materials. By analyzing complex data sets, simulating molecular interactions, and even predicting how substances will behave under different conditions, AI can accelerate the discovery process by leaps and bounds. We’re talking about breakthroughs like lighter, stronger alloys and more efficient solar cells, all designed by algorithms. So, yes, AI could very well design the next wonder material that revolutionizes industries across the board.
The Evolution of Material Science and Its Intersection with AI
From crafting rudimentary stone tools in the Paleolithic era to harnessing silicon for today’s high-tech industries, material science has served as the silent engine propelling human civilization forward.
This fascinating field specializes in transmuting raw elements into life-changing innovations—whether it’s ancient smiths refining iron into steel or modern-day engineers designing carbon-fiber composites for space exploration.
The pursuit of groundbreaking materials has been relentless, with each new discovery setting a higher standard for what’s possible. Yet, despite these monumental strides, the traditional approach to material science comes with inherent challenges.
Research in this area can be painstakingly slow, prohibitively expensive, and require a litany of trial-and-error experiments.
Scientists often find themselves constrained by the limits of human intuition and the available experimental techniques.
Computational methods have certainly eased some of these constraints. However, even these advanced systems often necessitate oversimplifications that don’t fully represent the intricate nature of real-world materials.
This is where AI can step up, helping to calculate and simulate, poised to tackle these complexities head-on and potentially revolutionize how we discover and develop the wonder materials of tomorrow.
For example, AI can supercharge the search for advanced battery materials. Traditionally, discovering a new material for batteries could involve years of laboratory testing. AI algorithms can analyze vast datasets of chemical properties and interactions in a fraction of the time, predicting which combinations are most likely to yield high energy storage and fast charging capabilities.
This fast-tracks the experimental phase, allowing scientists to focus their efforts on the most promising candidates, potentially bringing new battery technologies to market years sooner.
How AI Can Lead the Way
Enter artificial intelligence, the new workhorse in the area of material science. AI has the unique ability to analyze enormous datasets, way beyond human capability, to find patterns and correlations that were too obscure to put together.
Imagine trying to solve a Rubik’s Cube that has hundreds of colors and thousands of squares; this is the level of complexity we’re dealing with in material design. AI algorithms can run countless simulations in the time it takes a researcher to complete one lab experiment, drastically accelerating the pace of discovery.
Harnessing AI for Real-world Applications
Companies and research institutions are already employing AI to create new materials with real-world applications. From designing more efficient battery materials that charge in seconds to crafting new alloys for aircraft that are lighter yet stronger, the AI-designed substances are already trickling into various industries.
Researchers at the University of California San Diego have created an AI algorithm called M3GNet, which can predict the structure and characteristics of over 31 million materials that haven’t been made yet.
This tool has the potential to unearth materials with extraordinary properties. The team is already using M3GNet to rapidly generate a comprehensive database of theoretical materials, focusing on finding better electrodes for lithium-ion batteries used in devices like smartphones and electric cars.
Shyue Ping Ong, a nanoengineering professor at UC San Diego, likens M3GNet to AlphaFold, Google DeepMind’s revolutionary protein-structuring AI. The introduction of M3GNet and its associated database, matterverse.ai, could significantly broaden the scope of material exploration.
AI as a Collaborative Force
It’s worth noting that AI isn’t here to replace scientists but to work alongside them. Combining the computational power of AI with the nuanced understanding and creativity of human experts creates a formidable team. As AI systems learn from each round of simulations and human-guided experiments, they get better at predicting material behaviors, leading to more rapid and accurate discoveries.
AI has the potential to be the crucible in which the next generation of wonder materials are forged. It amplifies the possibilities of what we can achieve, smashing through barriers that once seemed insurmountable.
So, the question isn’t so much can AI create the next wonder material—it’s more like what wonder material will AI bring us next?
The Current State of Material Science: Limitations and Challenges
Material science has come a long way from the days when the alchemists sought to turn lead into gold. Today, we have a deep understanding of elements, molecules, and the intricate dances they perform to create everything from bulletproof vests to solar panels.
However, even with the most advanced tools and methodologies, material science is at a crossroads, grappling with limitations and challenges that slow its march towards the future.
The Slow Pace of Discovery
One of the most glaring issues is the snail-paced progression of research and development. The journey from a conceptual material to a market-ready product has traditionally been a marathon, not a sprint. Years, sometimes decades, are invested in the process of theoretical modeling, lab synthesis, testing, and finally, commercial scaling. But time is a luxury that critical sectors like healthcare and renewable energy simply can’t afford.
The Issue of Complexity
Materials are not standalone entities; they interact with their environment in complicated ways. The sheer number of variables, such as temperature, pressure, and chemical composition, make it difficult to predict how a new material will behave in a real-world situation.
Computational methods have been a boon, but they come with their own set of challenges, like the need for massive computational power and the risk of oversimplification.
Human-Limited Insight
Even the most brilliant scientists have cognitive limitations when it comes to processing complex data and multidimensional relationships.
Our traditional approach relies heavily on human intuition, honed through years of education and experience and we just don’t know what materials will be needed in the future. Yet, there’s a ceiling to what the human brain can compute, and thus, a limit to the speed and efficiency of the discovery process.
Environmental and Ethical Concerns
Let’s not forget the elephant in the room: sustainability. Many current materials, although high-performing, are not environmentally friendly. They may require rare elements, contribute to pollution, or be non-biodegradable, creating a slew of ethical and environmental issues that can’t be ignored as we face an environmental emergency.
Take acrylic, for example. When it ends up in landfill (which it too often does) it’s detrimental to the environment. It isn’t compostable or biodegradable, so it will persist within the ground for a very long time.
Economic Constraints
Research isn’t cheap. The cost of high-tech laboratories, advanced computational resources, and specialized personnel can quickly escalate, making material innovation a high-stakes gamble. Funding is often contingent on short-term returns, making it harder to invest in long-term, high-risk, high-reward projects.
With its capacity to process enormous datasets, make lightning-quick calculations, and model complex systems, AI is poised to shatter the barriers constraining contemporary material science.
What AI Brings to the Table in the Search for the Next Wonder Material
As we’ve seen, the current landscape of material science, though rich in promise, is littered with challenges. Next, we’ll explore the dazzling array of capabilities that AI brings to the table.
Big Data Analytics: Making Sense of Complex Material Properties
In the intricate world of material science, the devil is in the details—or more accurately, in the data. Massive volumes of data are generated through experiments, simulations, and real-world applications, creating a complex labyrinth that researchers must organize into something that can be put into action.
Traditional methods, while invaluable in the past, often struggle to manage and interpret these vast datasets. This is where Big Data Analytics, powered by artificial intelligence can step up to lead the way.
The Big Deal about Big Data
In a field that deals with countless variables like molecular structures, chemical compositions, and environmental conditions, the amount of data generated is simply mind-boggling. We’re talking petabytes of information, equivalent to continuous years worth of high-definition video. Parsing through this sea of data manually is akin to finding a needle in a haystack, except the haystack is the size of Mount Everest.
AI algorithms, specially designed for big data analytics, slice through these complexities with surgical precision. Machine learning techniques, from clustering to neural networks, can identify patterns and correlations that would be virtually impossible for humans to detect.
These algorithms act like a magnet, pulling out the “needles” from the data “haystack” with remarkable efficiency.
Simulation and Prediction
If Big Data Analytics is the gifted code-breaker of material science, then Simulation and Prediction are its visionary architects.
Picture this: You’re a scientist with a potentially groundbreaking idea for a new material, but the process of bringing it to life through experiments could take years if not decades. Now, what if an AI algorithm could simulate the entire lifecycle of that material in mere days, or even hours? This is where AI-driven simulations and predictions are dramatically accelerating the pace of discovery.
AI Virtual Experimentation
Traditionally, material scientists had to rely on a painstakingly slow iterative process: hypothesize, test, analyze, refine, and repeat. Each experiment involves laborious preparation, meticulous execution, and the patience of a saint.
On the flip side, AI algorithms can perform thousands of virtual experiments in the blink of an eye, navigating the multi-dimensional landscape of material properties to predict outcomes with astonishing accuracy.
AI Predictive Models
Utilizing machine learning algorithms, these models can predict a material’s behavior across various conditions—be it temperature, pressure, or any other critical factor—without even synthesizing the material.
For example, AI can simulate the stress and strain on an aerospace alloy, helping engineers understand its weakest link when under extreme conditions, all before it ever leaves the drawing board.
Accelerated Validation
With these simulations, scientists can quickly validate or discard hypotheses. This makes it possible to iterate and improve designs at warp speed. This rapid validation isn’t just about speeding up the process; it also means we can explore materials and combinations that were previously deemed too risky or time-consuming to work on.
These AI-powered simulations work best when paired with real-world experiments. The algorithm learns from each new set of data, refining its predictive capabilities in a virtuous feedback loop. It’s a synergistic relationship where AI and human expertise elevate each other, each iteration bringing us closer to that next wonder material.
If we take the case of battery technology, for instance. By sifting through countless combinations of materials, voltages, and chemical interactions, AI can pinpoint the optimal design for a battery that charges faster and lasts longer.
Similarly, in the medical field, AI can analyze a myriad of factors like biocompatibility, toxicity, and structural integrity to develop implants or prosthetics that will be much more likely to integrate seamlessly with human tissue.
Related Article: Can Artificial Intelligence Be Creative? (What You Need to Know)
How AI Works in Material Design
As we’ve looked at what AI can accomplish in material science, you might be wondering how it actually pulls off these feats of engineering and just how AI works in material design.
Algorithmic Frameworks Commonly Used: Neural Networks, Genetic Algorithms, and More
At the heart of how AI is revolutionizing material science are intricate algorithmic frameworks that act as the brainpower behind material design. Let’s jump into some of the big players in this computational ensemble: neural networks, genetic algorithms, and more.
Neural Networks: The Master Pattern-Recognizers
In a field replete with data and complex relationships, neural networks are like the Sherlock Holmes of algorithms. Inspired by the structure of the human brain, these networks are made up of layers of linked nodes that handle data processing and analysis.
They’re particularly adept at identifying patterns and learning from data. This makes them invaluable for tasks such as predicting material properties and understanding intricate chemical interactions.
Genetic Algorithms: The Evolutionary Artists
Nature has been an extraordinary material designer, churning out marvels like spider silk and nacre through millions of years of evolution. Genetic algorithms take inspiration from nature’s own evolutionary processes.
Starting with a ‘population’ of possible solutions, the algorithm selects the ‘fittest’ individuals based on specific criteria, then ‘mates’ them to produce a new generation. Over iterations, this Darwinian dance results in materials that meet or exceed desired properties.
Decision Trees and Random Forests: The Decision-Makers
When material scientists need a decisive yet nuanced analysis, they often turn to decision trees and their more robust cousins, random forests. These algorithms break down complex decisions into simpler, hierarchical questions, each branching out into more specific inquiries.
By analyzing a multitude of factors, they offer insights into which materials or combinations are the most promising for a given application.
Reinforcement Learning Algorithms: The Quick Learners
Imagine a virtual scientist who not only learns from its successes but also its failures. That’s what reinforcement learning algorithms do—they adapt based on the consequences of their actions. Used in applications like drug discovery and molecular design, these algorithms iteratively improve upon their choices, optimizing material properties with a dogged determination.
The algorithmic frameworks behind AI in material design are as diverse and specialized as the materials they help create. Each algorithm offers its own unique strengths, and it’s often the symphony of these algorithms working in concert that leads to the most groundbreaking discoveries.
Ethical and Environmental Considerations
How does the AI revolution impact ethical and environmental considerations? As we harness algorithms to create wonder materials, are we also pondering the ethical implications and the footprint we leave on our planet? Next, we’ll tackle these nuanced issues and look closer at the multifaceted responsibilities that come with this technological windfall.
Intellectual Property: Who Would Own an AI-Created Wonder Material?
When a groundbreaking material emerges from the labyrinth of algorithms and data, who gets to claim it? Is it the team of human scientists or the creators of the AI algorithm?
The Traditional Paradigm
Traditionally, IP rights for a new material would go to the inventors and the institutions they represent. Patents would be filed, and the fruits of human ingenuity would be legally protected for a number of years. But introduce AI into the mix, and the lines of ownership blur considerably.
A Shared Effort?
Some argue that AI is merely a tool wielded by human researchers, making the case for traditional IP rights straightforward. However, what happens when an AI algorithm discovers a material that human researchers had not even considered? The AI becomes more than just a tool; it becomes a collaborator. Should it, or its developers, get a share of the IP pie?
Legal Considerations
Countries are still grappling with these issues, and legislation is evolving. Some jurisdictions are considering recognizing AI as an “inventor” for the purpose of patents, although this comes with its own can of worms. For instance, if AI can own a patent, can it also be sued for infringement?
Ethical Considerations
Beyond the legal aspect, there’s an ethical dimension to consider. If a material has broad societal benefits, such as a new eco-friendly energy source, should its IP be more freely accessible? On the flip side, if an AI-designed material poses risks, who bears the ethical responsibility?
Sustainability: Can AI Design Environmentally Friendly Materials?
While the quest for innovation often captures the spotlight, there’s another hero quietly making waves in the AI-driven material science narrative: sustainability. With climate change and environmental degradation taking center stage in global conversations, the question arises—can AI algorithms don the green cape and design materials that are not only remarkable but also eco-friendly? The answer is a resounding yes, and here’s how.
The Green Algorithm
AI is perfectly suited to tackle the multivariate problem that is sustainability. By simultaneously optimizing for performance and environmental impact, AI algorithms can create materials that do more with less. We’re talking lightweight composites that require fewer raw materials, or biodegradable plastics that offer the same utility without the lasting environmental toll.
The Circular Economy
AI can also play a pivotal role in designing materials that fit within a circular economy—where the end of one product’s life is merely the beginning of another’s. Algorithms can predict how easy it is to recycle or repurpose materials, leading to designs that consider not just the material’s first act, but its entire lifecycle.
Real-world Impact
Companies are already capitalizing on AI’s green potential. Take Adidas’s Futurecraft.Strung shoe as an example. The shoe is made using a robot powered by AI algorithms that weave a shoe upper from a single yarn, minimizing waste and optimizing material usage.
Another example is the development of AI-designed catalysts that can accelerate chemical reactions, thus reducing energy consumption in industrial processes.
AI’s role in material science isn’t just about breaking records; it’s about setting new standards—standards that align with a vision for a more sustainable and responsible future. With every algorithm that churns out a green material, we’re not just advancing science; we’re also honoring our commitment to the planet. The potential here is not just revolutionary; it’s restorative.
Industry Implications of AI in Material Science
The profound impact of AI in material science is set to ripple across sectors, reshaping economies and perhaps even societal norms. Next, we’ll look at the industry implications of AI’s growing role in material design, from the seismic shifts in job markets to the new frontiers of competitiveness.
Sectors most likely to benefit
While AI’s influence in material science promises to be ubiquitous, some sectors stand on the brink of extraordinary transformations. From the skies above to the microcosms of cellular biology, let’s examine the industries where AI’s touch is poised to be nothing short of revolutionary.
Aerospace
Aerospace is an industry that demands materials with a delicate balance of strength, weight, and resilience. Here, AI’s capacity to sift through millions of combinations to find that golden formula is nothing short of a godsend.
We’re talking about materials that can withstand extreme temperatures and pressures while being light enough to make air travel more fuel-efficient. This isn’t just a win for the aerospace giants; it’s a leap for mankind’s cosmic ambitions.
Medicine: Tailored Treatments and Implants
Imagine a world where medical implants are as unique as the individuals who receive them. AI is enabling this personalized approach, designing materials optimized for individual biochemistry and pathology.
From AI-designed stents that reduce the risk of complications to prosthetic limbs with enhanced functionality, the implications for healthcare are staggering. The era of one-size-fits-all medicine is giving way to customized solutions that promise to improve quality of life.
Renewable Energy
If there’s one sector that’s in bad need of innovation, it’s renewable energy. The need for efficient solar cells, long-lasting batteries, and robust wind turbines is pressing.
AI’s capacity to create materials that can capture more sunlight or store more energy is invigorating the race toward a cleaner, more sustainable future. As we grapple with the existential threat of climate change, AI could very well be the catalyst for a green energy revolution.
Automotive
As electric vehicles become the new normal, the automotive industry is scrambling for batteries that are lighter, charge faster, and last longer.
AI-designed materials are fueling this shift, making electric vehicles not just an alternative but potentially superior to their fossil-fueled counterparts. The ripple effects extend to the entire transportation sector, heralding a new age of efficiency and sustainability.
Construction
With urbanization on the rise, the construction industry is under immense pressure to build quickly yet sustainably. AI can help design materials that are both durable and eco-friendly, revolutionizing how we think about infrastructure. Think self-healing concrete or insulation materials that drastically reduce energy consumption.
The Economic Impact
As with any transformative technology, AI’s burgeoning role in material science carries a double-edged economic sword—potential for job creation on one side, risk of job displacement on the other.
The Upside: New Professions and Specializations
As AI takes on more of the heavy lifting in research and development, new roles are emerging that require a blend of technical acumen and domain expertise.
These are jobs that didn’t even exist a decade ago—AI-Material Science Liaisons, Data Curators for Material Properties, or even Ethical Compliance Officers specializing in AI-driven research.
Additionally, the demand for experts who can build, maintain, and improve upon these complex AI systems is on a steep incline.
The Flip Side: Automation Anxiety
The looming shadow on this sunny outlook is the displacement caused by automation (which will far outweigh job creation).
Routine tasks, particularly those in quality control or basic material testing, are susceptible to automation. AI algorithms can perform these tasks more quickly and accurately than humans. While the impact might not be immediate, there are genuine fears.
One way to negate this economic turbulence lies in education and reskilling initiatives. Governments and industries will need to collaborate closely to ensure that as jobs become obsolete, pathways open up for individuals to transition into new roles. In the absence of such efforts, the economic divide between AI ‘haves’ and ‘have-nots’ will widen distressingly.
The Issues Facing AI’s Material Science Journey
While the AI-fueled future of material science appears dazzlingly bright, it’s important not to overlook the speed bumps and roadblocks that lie ahead. Yes, we’re advancing at an incredible pace, but let’s pump the brakes for a moment and consider the challenges—both technological and human—that could steer us off course.
The Question of How Accurate and Reliable AI is in Material Science
In the area of material science, precision isn’t just important; it’s imperative. An error in the design of an aerospace material or a medical implant could result in catastrophic failures. Therein lies one of AI’s Achilles heels: How reliable are the designs churned out by algorithms?
While AI can simulate and predict material behaviors, the results are only as good as the data they’re based on and the algorithms that process them. Even the most sophisticated neural networks are susceptible to errors and biases, making human oversight indispensable.
Human Factors: The Inertia of Tradition and Skill Gaps
There’s a noticeable resistance among some traditionalists in the field to fully embrace AI’s capabilities. Whether it’s skepticism about the reliability of AI-generated designs or an ingrained preference for tried-and-true methods, breaking through this cultural barrier will take time.
Then there’s the skill gap. The integration of AI into material science demands a new kind of literacy—one that combines a deep understanding of materials with proficiency in data analytics and machine learning. Institutions and industries must drive efforts to nurture this hybrid skill set, or risk falling behind in the global race.
The Question of Trust
Let’s not overlook the delicate matter of trust. As AI systems become more complex, they also become more opaque, leading to what is often referred to as “black box” algorithms.
When human experts can’t fully understand or interpret the decision-making process of an AI system, trust erodes. And in a field as critical as material science, trust is the bedrock upon which innovations are built and adopted.
The Road Ahead: Navigating the Future of AI in Material Science
The fusion of AI and material science is more than just a thrilling subplot in the chronicle of technological advancement; it’s an unfolding epic with its own set of heroes, cliffhangers, and potential plot twists. So, what should we be on the lookout for as we navigate the road ahead?
Upcoming Projects and Research
Keep your eyes peeled for multi-disciplinary collaborations—these are the crucibles where groundbreaking solutions often take shape. Joint ventures between tech giants, academic institutions, and industry leaders are already underway. For instance, projects focused on AI-designed superconductors for efficient energy transmission or next-gen medical implants promise to be game-changers.
Similarly, keep tabs on public-private partnerships aimed at sustainability. Governments are increasingly recognizing the urgency of leveraging AI for green material solutions, and any new announcements in this area are likely to be bellwethers for the field.
Indicators That AI is About to Create the Next Wonder Material
Wondering what signs could indicate that AI is indeed about to unveil the next wonder material? Here are some cues:
Peer-reviewed breakthroughs: Credible publications highlighting AI-designed materials with unprecedented properties will be a strong indicator that the field has matured.
- Patent activity: An uptick in patents filed for AI-created materials could suggest that theoretical advances are transitioning into practical, market-ready solutions.
- Industrial Adoption: When major players in sectors like aerospace, medicine, or renewable energy announce the adoption of AI-designed materials, that’s your sign that the technology has moved beyond the lab and into the real world.
- Investment Flows: Venture capital and public funding steering towards AI-driven material science projects signal confidence and promise in the technology.
- Regulatory Approvals: Keep an eye on agencies like the FDA or the European Medicines Agency for approvals of AI-designed medical materials. Such endorsements will be a massive green light for the industry.
The road ahead for AI in material science is laden with promise, but it’s also a pathway that demands scrutiny, agility and a dollop of cautious optimism. While the ultimate destination—a world enriched by wonder materials birthed by AI—is captivating, the journey itself will be laden with lessons, detours, and immeasurable excitement. And as we hurtle down this road, one thing’s for sure: things are only getting started.